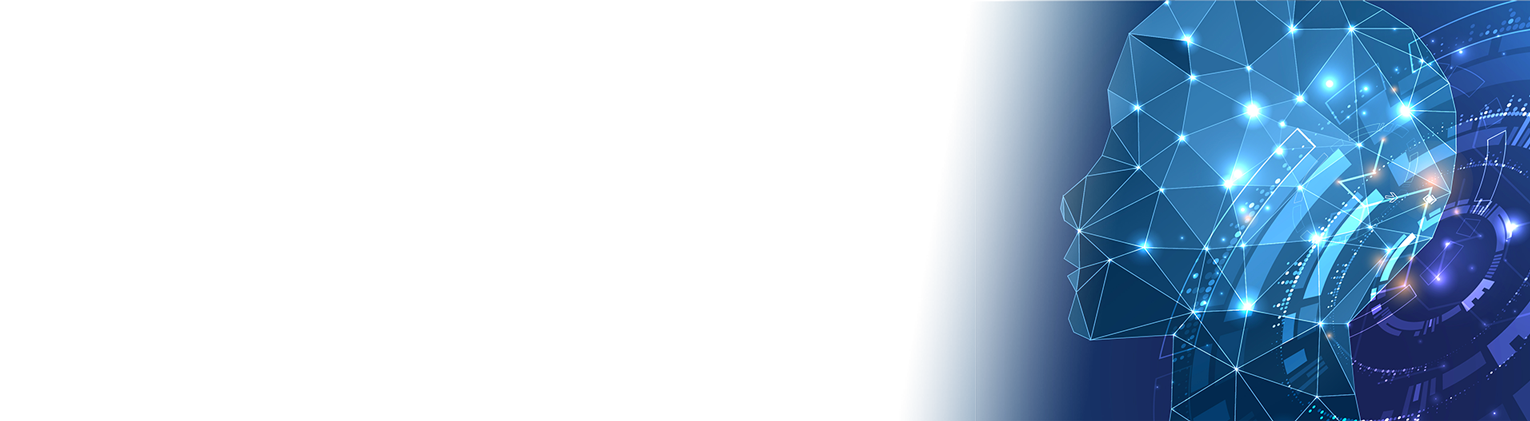
The power of the Valab® expert system in the medical laboratories
Valab®- Artificial Intelligence at the service of the medical laboratory validation specialist
A deterministic and open clinical expert system
Developed as a result of research in artificial intelligence applied to medical biology, Valab® uses an inference engine which executes automated verification rules.
Valab® is an expert system that is deterministic, reproducible, accountable and qualifiable, this sets it apart from self-learning systems of the "Deep Learning" and "Machine Learning" type.
For the same input data with the same parameter settings, Valab® will always give the same autoverification results.
To enhance Valab®'s autoverification power, users can create new rules, modify the preconfigured rule models, and create their own custom test rule models.
A cognitive approach
Human reasoning and behaviour is built up from a set of conscious (knowledge) and subconscious (experience) basic intellectual processes.
Developing an expert system involves:
- identifying all of the basic processes
- building a model capable of exploiting these processes
- validating the expertise model that is generated
The "intelligent" model at the origin of Valab® applies all of the processes we use to interpret a result according to a strategy that is close to human reasoning.
The acceptability of a result depends on a truly combinatorial approach and not on a classification in a pathological model.
The result does not depend on the application of an exclusive rule but, on the contrary, it depends on all of the applicable rules.
A practical example of a consistency check in medical report
Clinical Context
In the case of a patient with the clinical information "hepatitis" with a very abnormal ASAT result at 800 UI 37°, this information is compatible and it can be modeled with a simple rule.
However, if we add a normal ALAT result, at 20 UI 37°, it is clear that taking into account this new piece of information must make the overall picture incoherent. Only a combinatorial approach allows us to describe complex models in this way.
Valab®'s autoverification takes into account the following categories of information:
- Demographic: Age, gender
- Contextual: Prescriber, department, emergency, in-patient, etc.
- Medical: Therapeutic and clinical information (TCI)
- Technical: Complementary information (CI)
- Inter-parametric: Coherence of the results according to the correlation between the tests and the variation over time of all of the tests (inter-parametric kinetics)
The different steps in the autoverification strategy
The autoverification strategy to interpret the acceptability of each test result involves a number of steps:
Situate the context
First of all, to interpret the result it is necessary to situate:
- the value observed according to the demographics (age and gender), whether the value is normal, low or high. A haemoglobinaemia at 16 g/100 ml does not have the same significance for a man, a woman or a newborn baby,
- the current result in relation to a possible previous result: decrease-increase and improvement-deterioration.
Trigger all of the applicable rules for the previously analysed situation
- correlation rules for a result with no previous result (high values or low values)
- anteriority rules for a result with a previous result (upward variation or downward variation)
Each rule set comprises rules which:
- tend to validate (normally) abnormal results
- prevent the validation of (abnormally) normal results
Assess the overall acceptability of the result
A test can have a changing acceptability status according to its result or its variation over time:
- Validated
- Not validated for "Incoherence"
- Not validated for "Insufficient justification for an anomaly"
- Not validated for "Alert or critical value"
- Not validated for "Mandatory referral to validation specialist"
An easy and intuitive tool for autoverification rule modeling
Valab® includes an autoverification rule modeling tool which you can use to consult, modify or create your own rules with ease.
Example for tumor markers B2 Microglobulin:
Creating a demographic rules model
Creating a contextual rules model
Creating an inter-parametric rules model
Talk to our Sales Team
Find out more about the Valab® expert system, get advice on technical and medical biology questions and ISO 15189 accreditation.
+33 (0) 531 083 499
A deterministic and open clinical expert system
Developed as a result of research in artificial intelligence applied to medical biology, Valab® uses an inference engine which executes automated verification rules.
Valab® is an expert system that is deterministic, reproducible, accountable and qualifiable, this sets it apart from self-learning systems of the "Deep Learning" and "Machine Learning" type.
For the same input data with the same parameter settings, Valab® will always give the same autoverification results.
To enhance Valab®'s autoverification power, users can create new rules, modify the preconfigured rule models, and create their own custom test rule models.
A cognitive approach
Human reasoning and behaviour is built up from a set of conscious (knowledge) and subconscious (experience) basic intellectual processes.
Developing an expert system involves:
- identifying all of the basic processes
- building a model capable of exploiting these processes
- validating the expertise model that is generated
The "intelligent" model at the origin of Valab® applies all of the processes we use to interpret a result according to a strategy that is close to human reasoning.
The acceptability of a result depends on a truly combinatorial approach and not on a classification in a pathological model.
The result does not depend on the application of an exclusive rule but, on the contrary, it depends on all of the applicable rules.
A practical example of a consistency check in medical report
Clinical Context
In the case of a patient with the clinical information "hepatitis" with a very abnormal ASAT result at 800 UI 37°, this information is compatible and it can be modeled with a simple rule.
However, if we add a normal ALAT result, at 20 UI 37°, it is clear that taking into account this new piece of information must make the overall picture incoherent. Only a combinatorial approach allows us to describe complex models in this way.
Valab®'s autoverification takes into account the following categories of information:
- Demographic: Age, gender
- Contextual: Prescriber, department, emergency, in-patient, etc.
- Medical: Therapeutic and clinical information (TCI)
- Technical: Complementary information (CI)
- Inter-parametric: Coherence of the results according to the correlation between the tests and the variation over time of all of the tests (inter-parametric kinetics)
The different steps in the autoverification strategy
The autoverification strategy to interpret the acceptability of each test result involves a number of steps:
Situate the context
First of all, to interpret the result it is necessary to situate:
- the value observed according to the demographics (age and gender), whether the value is normal, low or high. A haemoglobinaemia at 16 g/100 ml does not have the same significance for a man, a woman or a newborn baby,
- the current result in relation to a possible previous result: decrease-increase and improvement-deterioration.
Trigger all of the applicable rules for the previously analysed situation
- correlation rules for a result with no previous result (high values or low values)
- anteriority rules for a result with a previous result (upward variation or downward variation)
Each rule set comprises rules which:
- tend to validate (normally) abnormal results
- prevent the validation of (abnormally) normal results
Assess the overall acceptability of the result
A test can have a changing acceptability status according to its result or its variation over time:
- Validated
- Not validated for "Incoherence"
- Not validated for "Insufficient justification for an anomaly"
- Not validated for "Alert or critical value"
- Not validated for "Mandatory referral to validation specialist"
An easy and intuitive tool for autoverification rule modeling
Valab® includes an autoverification rule modeling tool which you can use to consult, modify or create your own rules with ease.